The Over-Optimization of Everything
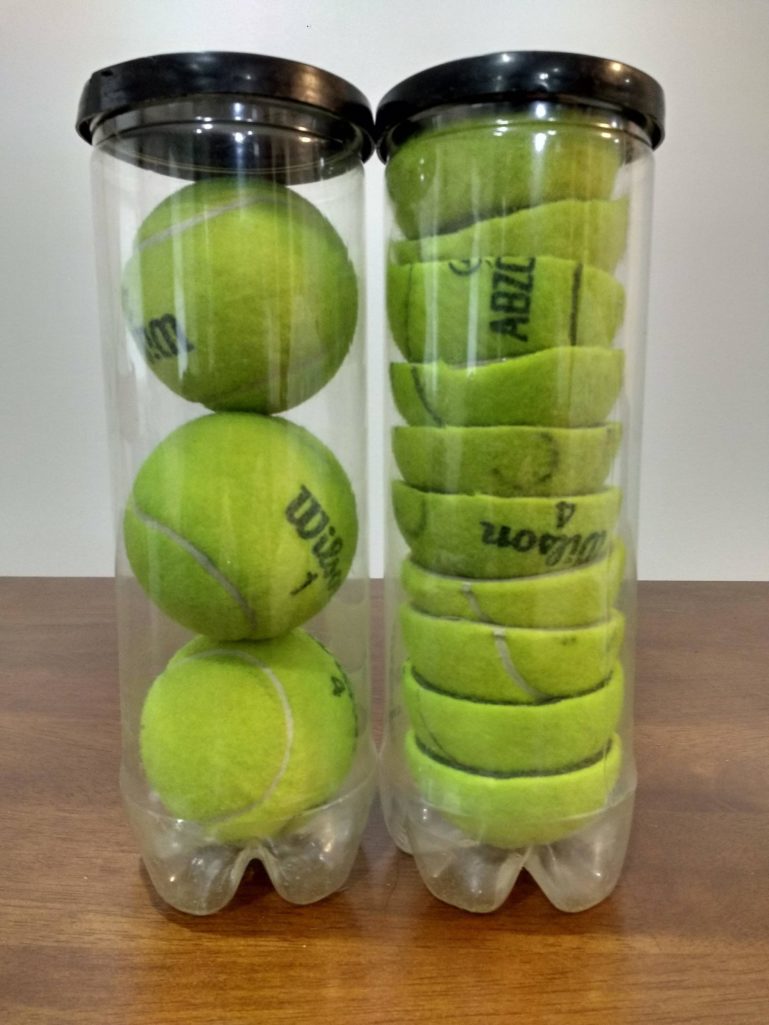
When I started my career in “data” back in 2010, the world was in the midst of an analytics revolution. The concept of “big data” was emerging into the mainstream, professional sports were starting to adopt analytics in new but not yet annoying ways, and the role of a data scientist was spreading beyond its roots at LinkedIn. It was also a field that was attracting folks who a decade earlier would have been more traditional “quants” on Wall Street or in private equity. Sure, the 2008 financial crisis may have had something to do with the latter, but nonetheless folks like myself who had options elsewhere (for me, a Computer Science grad working as a software engineer) were attracted to a field filled with the promise that technology now enabled the collection and processing of such volumes of data that decision making could not just be informed by data, but driven by it.
Looking back now, in 2022, at the last 15-20 years of analytics driven change I feel some respect for what we’ve accomplished, but mostly the realization that we’ve sucked some joy out of many corners of business, sports, travel, learning, and even our personal lives – all in the name of optimization.
The Ortiz Shift
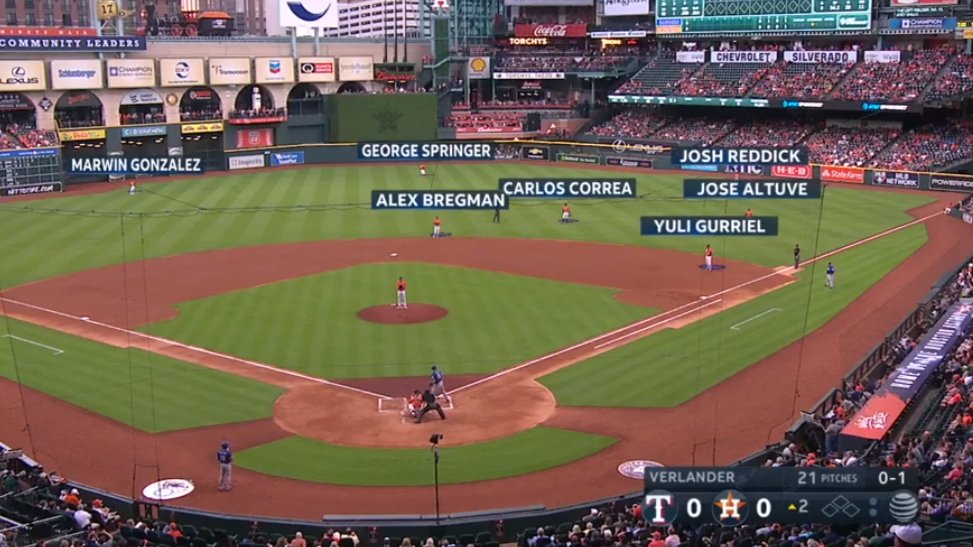
I grew up in Massachusetts. I also grew up a baseball fan. Thus, by law I’ve spent more time watching the Boston Red Sox than I’m willing to admit. Prior to 2006, as both a mediocre Little Leaguer and a long-time baseball fan, I never put much that much thought into defensive alignment, or “shifts”, as you often hear them refereed to (in vain in more recent years). I recall players shifting shallow or deep in the outfield depending on the power of a given hitter, and shifting left or right for a pull hitter on either side of the plate. On the infield, there were minor adjustment for pull hitters, playing in on the grass to prevent a bunt attempt or further back with runners on in hope of turning a double play. That was about it, and it was pretty uniform across teams and leagues.
Whether there was more happening than most fans realized prior to 2006, I’m not sure. But I am sure that 2006 was the year that defensive shifts got weird. I clearly recall the Red Sox playing the Tampa Bay Rays, and Ray’s manager Joe Madden essentially moving his entire infield to the right side of the field while facing David Ortiz. Ortiz was one of the top power hitters in the game, and as a lefty, he tended to pull the ball to the right-side of field. It was jarring, but it seemed to work. Thus, the “Ortiz Shift” was born, and the next 15+ years of baseball strategy shifted along with it.
Why was Joe Madden willing to throw out over a century of baseball strategy all of a sudden? Because in 2006 he was able to collect and analyze data like never before.
“I read the Einstein line that the definition of insanity is doing the same thing over and over again and expecting a different result,” says Maddon, 52, who pores over spray charts (showing the location of each ball that a batter puts in play) on his laptop computer. “So I thought it was time to do something different. Why not? The guy kills everybody, so let’s see if thisworks.”
Joe Madden on shifting for David Ortiz
None of this happened overnight. Baseball has been collecting data since the box score was first devised in 1858. The 1970s marked a major jump in baseball analytics with both the first known case of a computer simulation being used to make a lineup decision as well as the publication of the first The Bill James Baseball Abstract, and the furthering his concept of Sabermetrics, in 1977. Those roots grew into baseball front offices using analytics in new ways over the next few decades, reaching new heights when Oakland Athletics General Manager Billy Beane made Sabermetrics his framework for building his team starting in 2002. The analytical approach to roster decisions, and culture clashes that ensued, was popularized in Michal Lewis’ 2003 book, Moneyball which was later adapted into a wildly successful movie with Brad Pitt playing Beane. Talk about analytics going Hollywood. No wonder it was trendy to go into analytics at the time.
In the time since, something’s changed. Analytics have taken over almost every aspect of baseball. From the now dreaded shift, to players striking out at record rates in the interest of maximizing offense output, to teams being led not by baseball lifers, but MBAs and quants. With those changes have come pushback from fans. In the view of many, it’s made baseball even slower and more boring than even its worst critics painted it for years. Things have gotten so bad that Major League Baseball (MLB) is banning extreme shifts and instating a pitch clock to speed up the game starting in 2023. It’s been such a dramatic turn that Theo Epstein, an early analytics proponent but now MLB executive, not only supports the changes but has come to the same reckoning that I and many others have.
“The game on the field has been evolving for decades in a way that has taken us away from action, away from contact, away from a faster pace,” Epstein said. “And this is no fault of the players whatsoever. In fact, most of these trends have been driven simply by modernization, by data, and by front office optimizations. But the game has evolved in a way that nobody would have chosen if we were sitting down 25 years ago to chart a path toward the best version of baseball.”
M.L.B. Bans the Shift and Adds a Pitch Clock for 2023, NY Times, Sept. 9, 2022
A Personalized Bubble
I joined Facebook very early in its existence. Late 2004 to be exact. This was back when it was rolling out to various universities in waves, and you needed a specific .edu address to join. At the time I thought of it as a fun way to keep track of people I met at parties and in school and nothing more. By the time I was getting into analytics, Facebook had become a data giant. It was more clear to me then that it was a wealth of personal information, but as a user it didn’t bother me. I was pretty comfortable with targeted advertising online. I even worked in e-commerce, and was involved in personalizing marketing and advertising to potential customers.
Then in 2011, I read The Filter Bubble, by Eli Pariser and it stuck with me. I’m not here to claim its accuracy in predicting exactly where we stand today, but there’s no doubt that a personalized web & gated internet IS our reality. It’s no longer bold to claim that we’re shown ads, news, posts, pictures, and even misinformation based on the output of algorithms, all fed with the data exhaust trail we leave in our online lives. Given the financial impact of Apple limiting 3rd party data going to Facebook, it’s hard to argue that your experience on Facebook isn’t the product of a system doing its damnedest to understand your desires and turn them into clicks.
Setting aside the potential societal issues caused by all this, there’s another loss – the joy of serendipity on the internet. Anyone that’s worked in analytics knows the drill. Collect data, build model, score model, improve model. At risk of oversampling the work of us data folks, models in the online domain are judged based on their effectiveness on getting someone to do the thing our company wants them to do. It might be the click of a “Like” button, adding a product to our cart, scrolling a feed (and thus viewing ads) for a longer time than average, etc. By optimizing models based on engagement we naturally put content in front of people that we know they like or agree with. We’re essentially filtered into smaller and smaller groups, seeing what we “should” see.
While I appreciate the need to help me sort though endless content, there’s a big difference between a search engine answering a specific question I have, and YouTube sending me down a path of content that confirms my existing biases and attemps to take me to darker place. Sure, hand curated content and the time it takes to browse (can you remember browsing anything?) takes more of your time. It’s also not scalable for companies in the hunt to maximize profit. But it is a great way to find new interests, grow your world view, and generally have fun.
It’s one thing if our lack of true discovery ended when we got out into the fresh air, but think about the last time you explored a new city (or even your own city!) without the aid of your phone, and the personalized reviews and ads that come with it. Searching for a restaurant, and the results you get, may say as much about what you’ve done in the past as they do about the restaurants themselves.
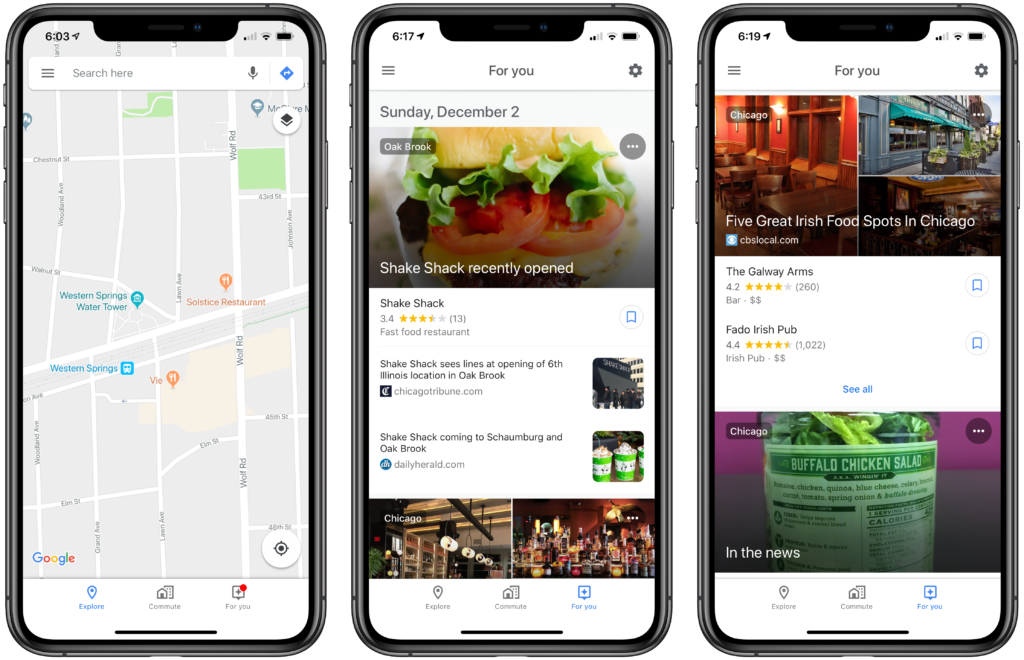
There is a ray of sunshine in the quest to personalize (and thus, optimize) your online experience. That is finding adjacent interests and content that crack open a new door of discovery. I appreciate something like Google Maps attempting to do that in the restaurant recommendation example, but I question whether their true goal of optimizing ad revenue equates to my personal joy of discovery. Rather than measuring success on the next click, measure it in months, and years of happiness and satisfaction. We have a lot of work to do in order to quantify such success, never mind expanding the acceptable time horizon for payoff. Still, I truly believe it’s worth the effort. Keep in mind all of the analytics challenges that felt impossible years ago, that are commonplace today.
Fit it All In
The cover image of this article is one of the best representations of an over-optimized life that I’ve ever seen. By cutting tennis balls in half, you can fit far more into a single container. But, given that the tennis balls are now useless for actually playing tennis, you wouldn’t do that, right? Well, I have news for you. We’re doing it all the time, thanks to the constant pressure to optimize our lives, and the data used to reinforce it.
Let me be clear. The desire to live and and work efficiently is not new. Just like baseball moved from box scores to extreme infield shifts over time, life optimization has gone from Benjamin Franklin sharing his musings, to your watch telling you when to stand up. It’s now also an app on your phone telling you that despite the fact you’re enjoying some quality family time that need 8 more minutes of exercise to keep your streak of green check marks alive. Though these innovations can truly be helpful, there’s now pressure to do the right thing 24×7. “What if I can squeeze in 6 more minutes of quality sleep tonight by turning this football game off early to keep my streak going?” may sound healthy, but at what cost?
Maggie Schuman, 32, is facing that very quandary now that her family is taking part in a Peloton challenge through the workout platform’s app.
“Every day everyone sends around a green check mark, and for some reason, now that I have that in my head of this thing I’m supposed to be doing, I’m not doing it,” Ms. Schuman, a product specialist in California, said. “I feel a bit like a failure.”
Stop Trying to Be Productive, NY Times, Updated July 13, 2020
When I was a software engineer, I recall dreaming in code some nights or even thinking in code as I walked through a store. Usually it was when I was focused on a particularly challenging programming problem or in a crunch at work. Now, whether it’s because I work in data or I’m just an average modern-day human, I find myself thinking about self-optimization in my sleep or in the back of my mind as I try to relax. “Should I go for a walk now, or in 20 minutes when my watch tells me the clouds will clear slightly?” “Is it worth leaving the house 10 minutes later and risking the stress of barely making my flight, or waiting at the airport of a bit longer? Google Maps predicts my trip will only take 32 minutes.”
Again, this is beyond old-fashion efficiency. The data needed, and predictive models required to power that forecast model or traffic prediction are all part of the analytics revolution that we’re taking part in. 20 years ago you might playfully pick on your father for leaving the airport hours early, but now you’re living in regret about arriving 22 minutes sooner than you needed to.
Like personalized content recommendations, I do appreciate some aspects of such information. I’m glad that Google Maps will reroute me before I hit gridlock that I can’t yet see. The same goes for the motivator of hitting fitness goals on my Apple Watch. Even more so in your life than in business however, perspective matters. Don’t let the algorithms rule your life and leave you with a badge for a new record of tennis ball debris in a container rather than a fun day of tennis with a friend.
Can We Go Back?
I believe that change leads to good outcomes in the long run, but not all change along the way is progress. The more I work in the data, the more I remind myself that just because something is technically possible doesn’t mean it’s the right thing to do. Not just ethically, but also in terms of what you’re optimizing for. In the examples discussed, there’s a fine balance between optimizing for a particular measure of success (getting a batter out, profit, minutes of sleep) and joy to all involved. It’s not only recommendation models that need to adjustment their goals, but us analytics professionals as well.
Major League Baseball cracking down on extreme defensive shifts is in reaction to fan disengagement (less joy), even at the cost of teams giving up more hits. Just because it’s possible to better defend against hitters, fans enjoy watching talented players dictate a winner rather than the team with the best analytics department.
While I don’t think we can go back to the way things were decades ago (nor do I think we should), I do believe that we can be more deliberate about how and when we use data. A “data informed” life will be far more of a life than a “data driven” one.