It Takes 3 Things to Get Value From Machine Learning
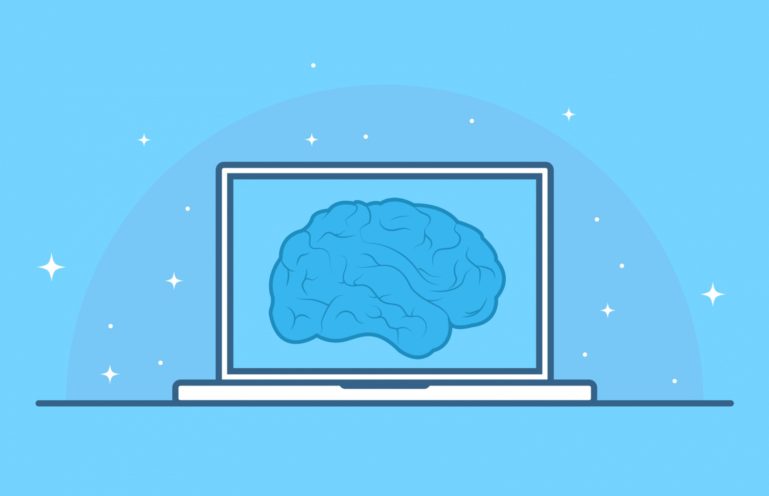
When I read about the $30 Million dollar series C funding raised by Crunchbase this morning, it reminded me of a common struggle I see in tech companies. That is, going from “machine learning is going to change our business” to actually using it to change their business.
In fact, there are 3 things that I believe it takes for a business to derive any meaningful value from machine learning (or AI, data science or whatever they brand it as) –
- They must have raw data that’s valuable.
- They must have a specific, strategic outcome in mind that is attainable with machine learning.
- They must be willing to invest in making it successful.
While that all sounds obvious, it’s incredibly difficult to get right. I see the struggle first hand often. Let’s take a look at Crunchbase as an example as to why it’s so hard.
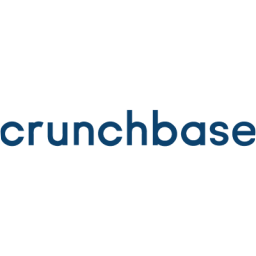
Valuable raw data
In numerous articles on the Crunchbase fundraise, machine learning and AI were headlined. That’s pretty common in fundraise headlines in the past few years, but in the case of Crunchbase it feels very authentic given that they’re in the business of collecting and making sense of data.
In fact, they have already monetized their data directly with their Crunchbase Pro product. They not only own valuable raw data, they have been able to structure and make sense of it to the extent it’s worth paying for. In other words, Crunchbase checks the first box.
A specific, strategic outcome in mind that is attainable with machine learning
In 2019, it’s not uncommon to have lots of raw data collected and stored. It’s also not uncommon for that data to have some potential value to machine learning models. What is a challenge is connecting the dots from that data to an outcome that is both of value to your customers and a good fit for machine learning.
In the case of Crunchbase, they have this part covered as well. Here’s a great quote from their CEO from the announcement per VentureBeat –
“One of the key technologies we’ll be investing in after this funding round is machine learning,” Crunchbase CEO Jager McConnell said in a statement. “Today, our users are already benefiting from powerful ML tools like Crunchbase Rank and Trend Score. In the near future, we’ll create personalized experiences that highlight companies our users should consider. These recommendations will be based on the type of companies they’ve engaged with previously, and their specific business use case, whether that’s sales prospects, partnership prospects, investment prospects, or some other B2B connection. Great machine learning relies on massive amounts of data to be effective — with Crunchbase’s private company data platform, we believe we’re uniquely positioned to harness the power of ML to help our users find the best new opportunities for them.”
The types of problems Crunchbase is planning to solve using machine learning are well worn paths – recommendations, personalization, scoring metrics, etc. This isn’t just some CEO buzz. I imagine the data team at Crunchbase is either already working on those things, or will be well on their way with some additional funds.
Be willing to invest in making it successful
This is where things go wrong for most companies. Though the work it takes to get the first two item right is worth celebrating, it’s nothing but press releases unless a company is willing to invest in executing.
The first obvious step is being willing to invest in talented people. Hiring data engineers, data scientists and machine learning engineers isn’t cheap. Organizations without a dedicated data team might feel they can utilize existing members of the engineering team to pull it off. I’ve never seen that work. You must being willing to invest in the talent specific to making machine learning projects successful.
The next step is to get buy-in from all the teams involved in making a commercial machine learning project successful. Let’s say that the Crunchbase data team builds an amazing personalization model, but the Product team has prioritized a revamped user-experience. Perhaps the Engineering team is busy rebuilding the backend of the Webapp and doesn’t have the bandwidth to help get the model into production. Other teams like Marketing are needed to publicize and “sell” the new features to customers. It’s a team effort!
It’s easy to say that Crunchbase has this covered as well, but that’s not necessarily the case. Sure, they just raised $30M which is nothing to sneeze at, but if you dig through the press release you’ll notice they call out other priorities as well. For example, an “All-New Look & Feel”, an “Improved API” and more. Those are all things that will require resources from the Engineering, Product and Marketing teams.
Will It Work?
I’m not in a position to know what’s going on inside of Crunchbase, so it’s impossible for me to say if they will be successful in delivering upon the promise of leveraging machine learning. Given that data has always been fundamental to their business, I think they’re more likely that most but it’s no sure thing. Personally, I think Crunchbase is a valuable resource and I hope it thrives.
What This Means for Your Organization
While Crunchbase is one specific example, the 3 keys to getting value from machine learning is universal. Many organizations struggle with one of the first two and never have a chance to fail on the 3rd. The “lucky” ones fail on the 3rd and are able to course-correct before it’s too late. It’s quite rare to see a company get it all right the first time, though I believe that as data science and machine learning processes mature we’ll see similar success rates that we do for software engineering today.
I think the lesson worth taking away is that the gap between aspiring to use machine learning and actually using it to generate revenue is bigger than the headlines make it sound. Don’t fall into the trap of overestimating what it can do without understanding the costs involved in making it successful.
Cover image courtesy of https://pixabay.com/users/mohamed_hassan-5229782/